Today, companies want to fully leverage machine learning to gain valuable insights, but they are increasingly constrained by privacy regulations and concerns related to the exposure of sensitive data. In response to these needs, we have designed HEDEL: Homomorphically Encrypted Distributed Ensemble Learning.
This new architecture, based on homomorphic encryption and distributed learning, enables the training of machine learning models directly on encrypted data, without ever accessing the data in plaintext. And it does so in an efficient, secure, and collaborative way. The scientific work (paper) based on HEDEL has been accepted by the scientific committee to be presented at the 2025 International Joint Conference on Neural Networks (IJCNN), which will take place in Rome from June 30 to July 5, 2025.
What makes HEDEL special?
HEDEL combines two key technologies:
Transfer Learning: This allows adapting an already trained model to new data. It drastically reduces the computational cost of training, as it requires only a few epochs to reach high performance.
Multi-Key Homomorphic Encryption (MKHE): This enables multiple participants to share encrypted data and models while maintaining strict control over access and use. Each participant, in fact, can stop the use of the data/models they share at any time.
The combination of these techniques had never been proposed before in the literature and represents an important scientific achievement reached by Dhiria.
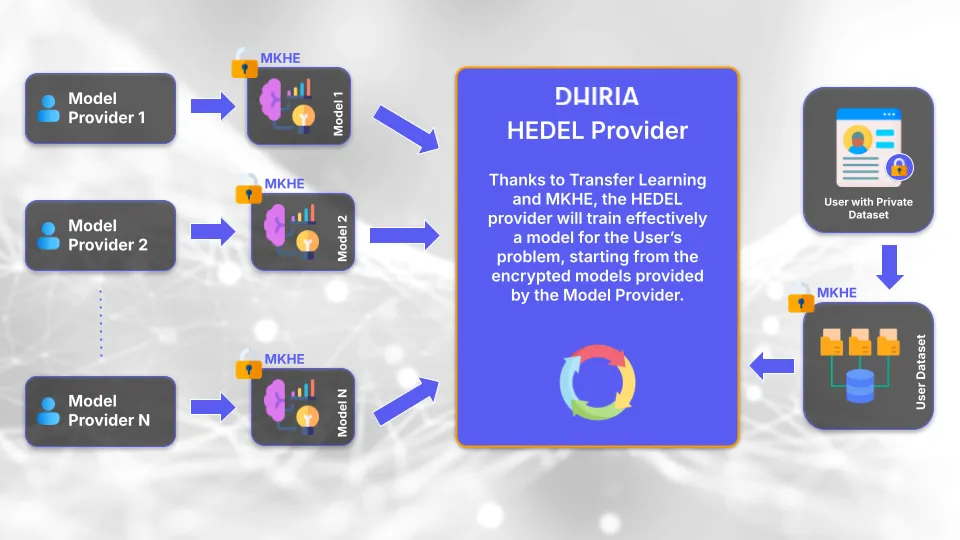
Why should companies be interested in HEDEL?
HEDEL is designed for scenarios where multiple players want to collaborate without exposing sensitive data. Collaboration is essential, especially when data is scarce; in these cases, collaboration ensures that enough data is available to obtain high-quality predictions.
Here are some concrete applications:
1. Healthcare Sector: Collaborative Predictive Diagnosis
A hospital can train a predictive model using the encrypted data of its patients and then combine it with models provided by other clinics (also encrypted). No institution shares plaintext data, but all benefit from a more accurate global model. Perfect for multi-center clinical research or public-private cooperation scenarios.
2. Banking and Fintech: Privacy-Proof Fraud Detection
Imagine several banks collaborating to detect suspicious activities. With HEDEL, they can train an anti-fraud system by combining their expertise (models) and encrypted customer data, without ever sharing sensitive information. An important advantage in this case is also the protection of the intellectual property of the anti-fraud models, which are not openly accessible to everyone but only as long as each participant gives active consent.
3. Manufacturing Industry: Federated Predictive Maintenance
Various industrial equipment suppliers can share predictive models to identify faults and anomalies in machinery, while the data collected in factories remains encrypted and confidential. This approach enhances the sector’s collective intelligence, reducing machine downtime for all without compromising confidentiality.
HEDEL represents a breakthrough in how we think about machine learning in sensitive contexts. It enables new forms of collaboration between companies, while ensuring:
Maximum respect for privacy
Protection of intellectual property
Good predictive accuracy
If your company works with sensitive data and is looking for solutions to fully exploit machine learning without compromising on security, HEDEL is a technology to adopt.